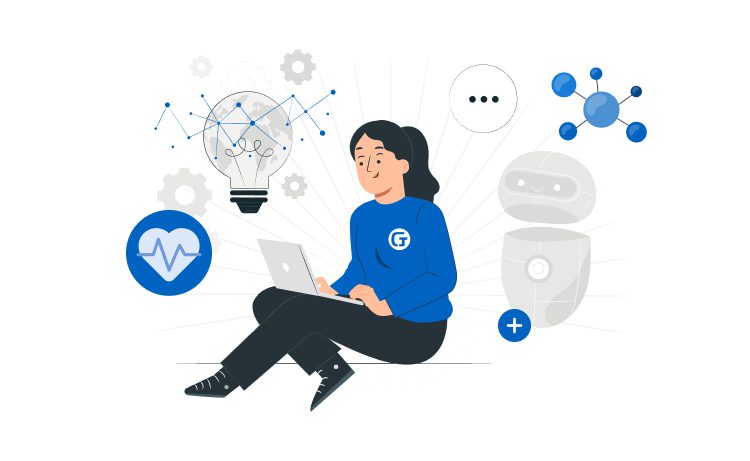
The Benefits of Machine Learning in Healthcare SaaS
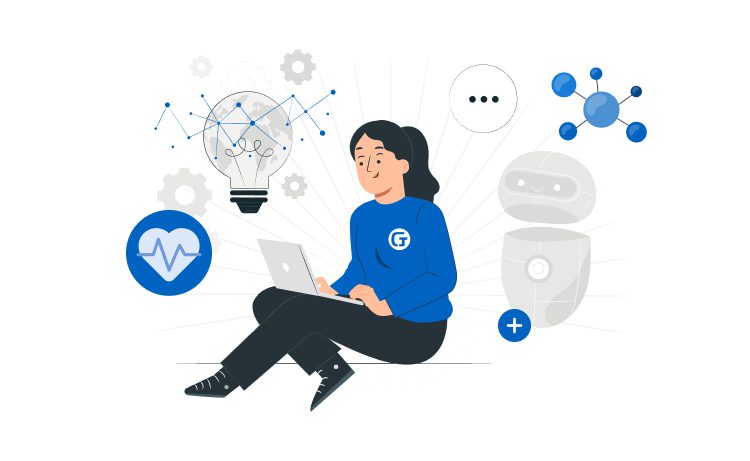
The intersection of technology and medicine has given rise to a groundbreaking convergence in healthcare we know as machine learning. Representing a transformative force, machine learning has the potential to revolutionize patient care, diagnosis, treatment, and overall healthcare operations.
In this article, we will explore the workings of machine learning in healthcare, the crucial role of data, and the broader spectrum of Artificial Intelligence (AI) in shaping the future of healthcare Software as a Service (SaaS).
Content
In the healthcare industry, artificial machine learning and AI are closely related concepts but have distinct roles.
AI refers to the broader field of creating intelligent machines capable of performing tasks that typically require human intelligence, such as problem-solving and decision-making.
Machine learning, on the other hand, is a subset of AI that focuses on enabling machines to learn from data and improve their performance over time without explicit programming.
In healthcare, AI encompasses a range of applications, including robotics, natural language processing, and expert systems, while machine learning specifically involves algorithms that can identify patterns in medical data, aiding in tasks like diagnosis, predictive modeling, and personalized treatment recommendations. If you want to learn more about the potential of AI in healthcare, make sure to check out this article.
Simply put, AI is the overarching concept, while machine learning is a specific approach within the broader spectrum of AI technologies applied in healthcare.
At its core, machine learning in healthcare involves using algorithms and statistical models to analyze vast amounts of medical data, facilitating better decision-making and personalized healthcare solutions.
Machine learning algorithms, a subset of AI, operate by analyzing large datasets to identify patterns, make predictions, and optimize decision-making processes. In healthcare, these algorithms are fed with patient data to extract meaningful insights, ultimately aiding in better medical decisions. These algorithms continuously learn and adapt from the data they process, enhancing their performance over time.
Machine learning algorithms are classified into different types, including supervised, unsupervised, and reinforcement learning, each finding unique applications in healthcare.
For instance, supervised learning is widely used for disease classification, while unsupervised learning is applied in clustering patient data for personalized treatment recommendations. Check out this article for more reasons to implement machine learning in healthcare and case studies of the companies who did.
Data is the lifeblood of machine learning in healthcare. Accurate and diverse datasets enable algorithms to learn, adapt, and continuously improve performance.
Now, thereโs a tricky part: making sure this data is safe and private. We need rules (like HIPAA) to keep your personal info under lock and key. Also, itโs like making sure our superhero team is diverseโour data needs to represent everyone, or else our machine friend might end up making biased decisions.
Another cool thing is how experts tag or label data, especially in things like medical images or records. Itโs like giving hints to our machine buddy about whatโs what. And just like we update our apps for the latest features, we need to keep our data fresh with the newest medical info.
Electronic health records (EHRs), medical imaging, genomics, and data from wearable devices are primary sources fueling machine learning models in healthcare. The success of machine learning applications hinges on data quality, quantity, and diversity.
AI in healthcare extends beyond predictive analytics and diagnosis, encompassing various technologies. According to Data Bridge Market Research, the artificial intelligence in healthcare market, which is USD 9.64 billion in 2022, is expected to reach USD 272.91 billion by 2030, at a CAGR of 51.87% during the forecast period 2023 to 2030.. The synergy of AI and machine learning is reshaping healthcare, making it more efficient, accurate, and patient-centric.
If you want to learn more about the potential of AI in healthcare, check out this article on our blog. Itโs been quite popular lately!
Machine learning applications in healthcare usher in a new era of innovation and transformation. These applications leverage algorithms and data-driven insights to provide healthcare professionals with powerful tools for diagnosis, treatment, and patient care.
According to Global Market Insights, disease diagnosis powered by AI and machine learning is projected to experience a growth exceeding 40%, surpassing the significant milestone of USD 2.5 billion by 2024.
Machine learning models can analyze patient data to identify early signs of cancer, diabetes, and heart conditions, significantly improving treatment outcomes and saving lives. Algorithms can examine medical images like X-rays and MRIs to identify anomalies indicative of diseases, demonstrating the potential of early detection.
Machine learning also plays an essential role in predictive analytics, forecasting disease trends, patient outcomes, and even the progression of chronic illnesses. This proactive approach empowers healthcare institutions to take preventive measures, ultimately improving overall patient care and safety.
Machine learning accelerates drug discovery by predicting the effectiveness of potential compounds and optimizing their chemical structures.
This technology significantly reduces the time and cost of drug development by simulating and predicting interactions between molecules. Additionally, machine learning aids in repurposing existing drugs for new applications, identifying treatments for previously untreatable diseases.
Machine learning enables healthcare providers to tailor treatment plans based on individual characteristics and medical history. This personalized approach results in more effective treatments and fewer adverse effects.
For instance, machine learning can analyze a patientโs genetic information to determine the most suitable medication and dosage, reducing the risk of adverse reactions. Customized interventions extend to rehabilitation programs, dietary recommendations, and lifestyle modifications, leading to better patient outcomes.
Machine learning plays a crucial role in optimizing healthcare operations. Predictive models can forecast patient admissions, allowing hospitals to allocate resources efficiently. AI-driven chatbots and virtual assistants automate administrative tasks, reducing costs and improving the patient experience.
Machine learning offers numerous benefits, transforming patient care and healthcare operations through enhanced accuracy, cost reduction, personalized care, and predictive capabilities.
Machine learning algorithms process vast amounts of data quickly and accurately, leading to more precise diagnoses and treatment recommendations.
For example, machine learning models trained on medical images can identify subtle abnormalities missed by human radiologists, enhancing the overall quality of care delivered to patients.
Efficiency gains from machine learning contribute to substantial cost reductions in healthcare. Predictive analytics can help hospitals optimize bed utilization, reduce emergency room wait times, and better allocate resources, ultimately lowering operational costs.
In addition, machine learning aids in identifying fraudulent insurance claims and optimizing supply chain management for pharmaceutical companies, positively impacting the affordability of healthcare services.
Tailoring treatments to individual patients improves outcomes and enhances patient satisfaction. Machine learning allows healthcare providers to deliver care aligned with patientsโ unique needs and preferences. This approach fosters patient engagement and adherence to treatment plans.
Machine learningโs ability to analyze large data sets and identify patterns makes it invaluable for predictive healthcare. Predictive models foresee disease outbreaks, patient readmissions, and equipment failures, empowering healthcare institutions to take preventive measures.
For example, predictive analytics can help public health agencies monitor and respond to disease outbreaks in real time, a critical capability in managing infectious diseases and ensuring public health.
Despite its transformative potential, machine learning in healthcare faces challenges such as data privacy concerns, lack of interoperability, bias, and the need for increased training and education.
When developing healthcare software with machine learning, prioritize data privacy and security. Utilize strong encryption for data, implement strict access controls, and employ anonymization techniques.
Adhere to industry regulations like HIPAA, conduct regular security audits, and keep your team informed about emerging threats. Transparently communicate data usage policies to users, obtain explicit consent, and empower users to manage their privacy settings. This holistic approach ensures a robust defense against potential breaches and fosters user trust in your healthcare software.
Diverse data formats and standards in healthcare systems hinder interoperability. Machine learning solutions must seamlessly integrate with various systems to be effective, promoting the exchange of patient information between healthcare providers.
Machine learning models can inherit biases from training data, leading to unfair treatment of certain patient groups. To mitigate bias, healthcare organizations should curate diverse and representative training datasets, employing fairness-aware machine learning techniques and transparent models.
Healthcare professionals need training to understand and use machine learning tools effectively. Bridging the skill gap between technology and healthcare is essential, requiring continuous learning and collaboration between healthcare and technology experts.
Future trends and innovations in healthcare machine learning include Explainable AI (XAI), quantum computing, blockchain, and telemedicine.
XAI seeks to make machine learning models more transparent and interpretable, providing clinicians with insights into AI-driven recommendations. This transparency enhances trust and adoption of AI-driven insights, particularly in critical healthcare applications such as radiology and pathology.
We have a great article on how to implement AI in Healthcare SAAS Products. Check it out!
Quantum computing holds immense potential for solving complex healthcare problems, accelerating drug discovery, genomics, and optimization of treatment plans. Its ability to process vast amounts of data and perform complex calculations at unprecedented speeds makes it a transformative force in healthcare.
Blockchain technology enhances healthcare data security, ensuring integrity and patient control over medical records. The decentralized approach allows patients to grant and revoke access to their data, improving trust and traceability of pharmaceuticals in the supply chain.
The fusion of telemedicine and AI expands access to healthcare services, particularly in remote areas. AI-driven chatbots and virtual health assistants provide instant medical advice, facilitating remote consultations, diagnosis, and patient monitoring.
When using telemedicine AI in healthcare apps, aim for user-friendliness, accuracy, and privacy. Check those algorithms, lock down data security, and keep things up-to-date. Make sure your AI talks to other healthcare systems smoothly, and get the experts involved for a top-notch experience. The Glorium team is here for you with any assistance needed!
Dmitriy StepanovCo-Founder & CTO at Glorium Technologies
Glorium Technologies stands at the forefront of innovation in the development of machine learning and AI-backed digital healthcare products. With a dedicated team of experts, we bring a wealth of knowledge and experience to the intersection of technology and healthcare.
Our proficiency lies in harnessing the power of machine learning algorithms to analyze vast datasets, facilitating accurate disease diagnosis, predictive analytics, and personalized treatment plans. We understand the pivotal role of data in healthcare, ensuring its privacy and diversity while adhering to regulations such as HIPAA.
Our expertise extends beyond traditional applications, encompassing the optimization of healthcare operations through predictive models, AI-driven chatbots, and virtual assistants. We take pride in our ability to address challenges such as data privacy concerns, bias mitigation, and the skill gap through continuous learning and collaboration.
As a forward-thinking company, Glorium Technologies is poised to lead the way in future trends, incorporating Explainable AI, quantum computing, blockchain, and the integration of telemedicine to create cutting-edge solutions that revolutionize the landscape of digital healthcare.
Machine learning in healthcare SaaS represents a transformative force with the potential to reshape patient care, diagnosis, treatment, and healthcare operations. Its benefits, challenges, and future innovations highlight the need for responsible implementation and continuous refinement.
Embracing these technological advancements while addressing associated challenges will be key to realizing the full potential of machine learning in healthcare. As the industry evolves, collaborations, training initiatives, and responsible practices will ensure that machine learning contributes positively to the future of healthcare.
AI encompasses a broader range of technologies aiming to create intelligent machines, while machine learning is a subset of AI specifically focused on enabling machines to learn from data.
In healthcare SaaS, AI can involve applications like robotics and natural language processing, whereas machine learning applies algorithms to analyze medical data for tasks such as diagnosis and predictive modeling.
Machine learning algorithms in healthcare analyze large datasets to identify patterns, make predictions, and optimize decision-making. Common types include supervised learning for disease classification, unsupervised learning for clustering patient data, and reinforcement learning for various applications.
These algorithms continuously learn and adapt from the data they process, improving their performance over time.
Data is crucial for machine learning in healthcare, serving as the foundation for algorithms to learn and adapt. Accurate, diverse, and secure datasets, including electronic health records, medical imaging, and genomics, are essential for the success of machine learning applications.
Data quality ensures that algorithms provide meaningful insights and predictions while adhering to privacy regulations such as HIPAA.
Machine learning offers benefits such as improved accuracy and efficiency in diagnosis, cost reduction through operational optimizations, personalized patient care based on individual characteristics, and predictive capabilities for disease outbreaks and patient outcomes.
These advancements enhance the overall quality of patient care while making healthcare operations more efficient and cost-effective.
Challenges include data privacy concerns, lack of interoperability, bias in machine learning models, and the need for increased training and education for healthcare professionals.
Addressing these challenges requires robust encryption and access controls for data privacy, efforts to enhance interoperability, the use of diverse and representative training datasets to mitigate bias, and ongoing training initiatives to bridge the skill gap in healthcare.